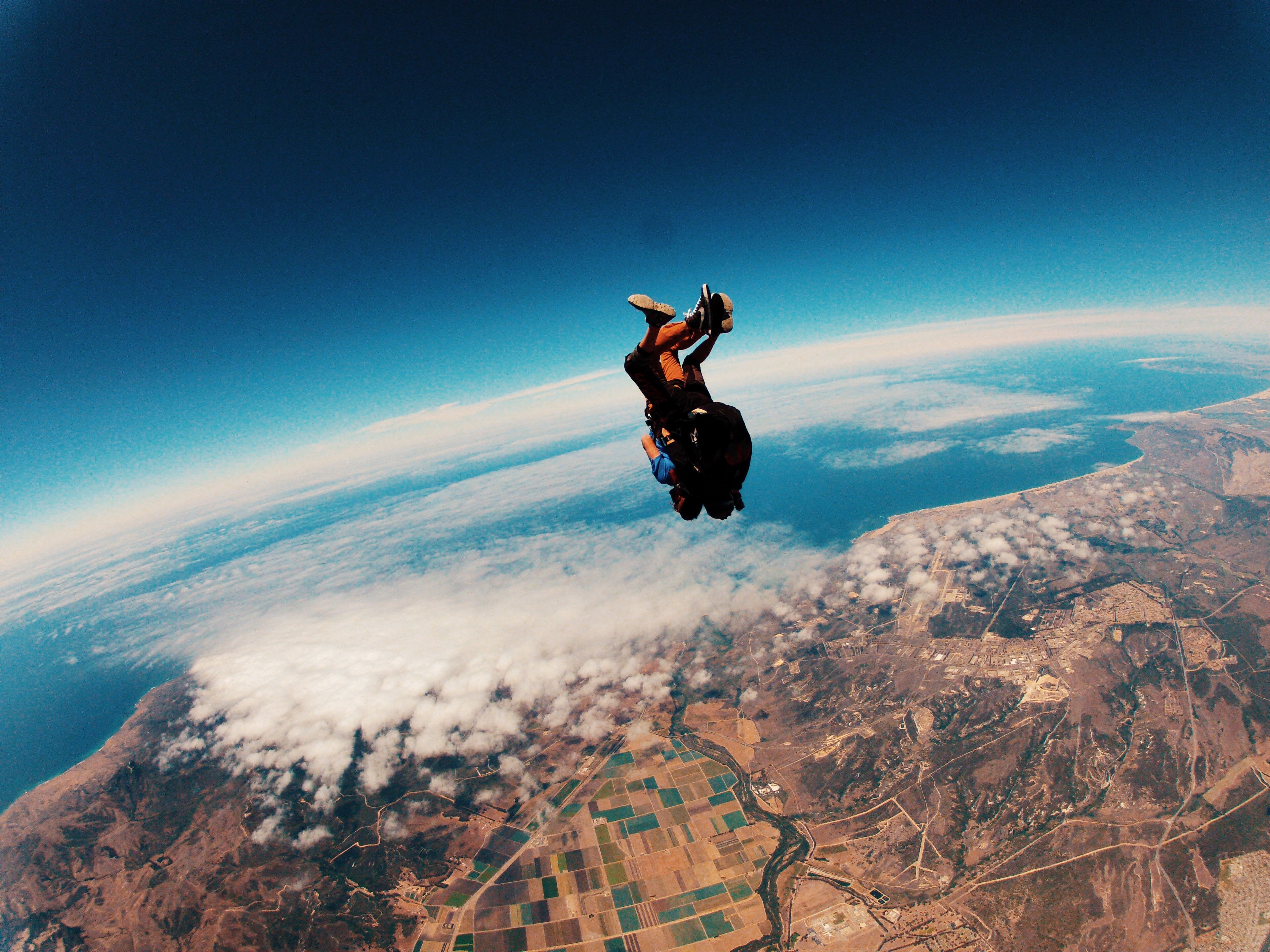
Gravitational Attraction
What would happen if two people out in space a few meters apart, abandoned by their spacecraft, decided to wait until gravity pulled them together? My initial thought was that …
This article re-examines the prior probability of Jesus mythicism, recently put forward by Richard Carrier. Carrier argues that this probability is relatively high because Jesus is among the so-called "Rank-Raglan heroes" from Mediterranean Antiquity, who are all fictional. If Jesus was the only historical example, he would be exceptional, which is inherently improbable. We make use of a recent survey of primary sources which has identified a large number of additional historical ancient Mediterranean Rank-Raglan heroes and draw attention to a peculiar feature of these figures which appears, at face value, highly relevant to the question of their historicity. This is the dramatic date, or the date when the person in question is depicted as living. We show that all ancient Mediterranean Rank-Raglan heroes depicted as living in very distant past are fictional while almost all living in a relatively recent past, much closer to the dramatic date of Jesus, are historical. We anticipate several potential objections to this finding, fully reproduce Carrier's formal Bayesian analysis and show that when the dramatic date of these newly identified heroes is taken into account, the prior probability of Jesus mythicism becomes very small
This article re-examines the argument from name popularity appearing in the 'Palestinian Jewish Names' chapter of Richard Bauckham's Jesus and the Eyewitnesses in light of patterns of the name popularity found in extra-biblical texts. Bauckham postulates that if Gospels-Acts contained named characters invented in the process of anonymous community transmission, the statistical distribution of name popularity in Gospels-Acts would not align well with the distribution in the ancient Palestinian Jewish population, particularly if the invention took place in the Diaspora. We test this by analyzing the name popularity in two corpora of texts that contain many recognizably fictitious first-century Palestinian Jewish characters, namely a corpus of Christian extra-biblical works consisting of the Clementine Homilies, the Acts of Pilate, and Solomon of Bosra's Book of the Bee, and the corpus of the Babylonian Talmud. Contra Bauckham, name popularity distributions in these two corpora do not correspond to the distribution among first-century Palestinian Jews statistically significantly worse than the distribution in Gospels-Acts. Moreover, the two corpora paradoxically align better in some respects because they do not exhibit a disproportional representation of specific names observed in Gospels-Acts.
In Jesus and the Eyewitnesses, Richard Bauckham argues that the popularity of personal names in Gospels-Acts corresponds remarkably well to name popularity among late ancient Palestinian Jews and that this can only be the case if Gospels-Acts characters are in most cases historical as opposed to invented in the process of 'anonymous community transmission'. Unlike the rest of the book, this argument has almost entirely evaded scholarly scrutiny. We re-examine Bauckham's conclusions, asserted with a remarkably high level of confidence but almost entirely without an actual statistical evaluation of his onomastic data, and perform the appropriate statistical analysis on the most recent dataset of Palestinian Jewish male names from 4 BCE to 73 CE. We show that Bauckham's thesis of the Gospels being based on eyewitness testimony offers no advantage in explaining the observed correspondence between name popularity in Gospels-Acts and in the contemporary Palestinian Jewish population over alternative models of name assignment, which Bauckham is meant to refute. This is because the sample of Gospels-Acts characters that are in contention of being invented in the first place is relatively small. This prevents any strong conclusions for or against Bauckham's thesis from being drawn based on the limited Gospels-Acts data. Moreover, our statistical analysis identifies some, albeit weak, evidence against Bauckham's thesis.
Purpose: In this work we propose an implementation of the Bienenstock-Cooper-Munro (BCM) model, obtained by a combination of the classical framework and modern deep learning methodologies. The BCM model remains one of the most promising approaches to model the synaptic plasticity of neurons, but its application has remained mainly confined to neuroscience simulations and few applications in data science. Methods To improve the convergence efficiency of the BCM model, we combine the original plasticity rule with the optimization tools of modern deep learning. By numerical simulation on standard benchmark datasets, we prove the efficiency of the BCM model in learning, memorization capacity, and feature extraction. Results In all the numerical simulations, the visualization of neuronal synaptic weights confirms the memorization of a human-interpretable subsets of patterns. We numerically prove that the selectivity obtained by BCM neurons is indicative of an internal feature extraction procedure, useful for patterns clustering and classification. The introduction of competitiveness between neurons in the same BCM network allows the network to modulate the memorization capacity of the model and the consequent model selectivity. Conclusion The proposed improvements make the BCM model a suitable alternative to standard machine learning techniques for both feature selection and classification tasks.
In a series of previous studies, we provided a stochastic description of a theory of synaptic plasticity. This theory, called BCM from the names of the three authors, has been formulated in two ways: the original formulation, where the plasticity threshold is defined as the square of the time-averaged neuronal activity, and a newer formulation, where the plasticity threshold is defined as the time average of the square of the neuronal activity. The newest formulation of the BCM rule of synaptic activity has interesting statistical properties, derived from a risk (or energy) function, the minimization of which leads to seeking of interesting projections in high-dimensional space. Moreover, these two rules, if implemented by a chemical master equation approach, show another interesting difference: the original rule satisfies the detailed balance, whereas the other not. Based on this different behavior, we found a continuous parameterization between these two rules. This parameterization shows a minimum that corresponds to maximum negative eigenvalues of the Jacobian matrix. In addition, the newest rule, due to the fact that it is in a nonequilibrium steady state (NESS), shows a higher level of plasticity than the original rule. This higher level of plasticity has to be interpreted in the framework of open thermodynamical systems and we show that entropy production and energy consumption in the newest rule are both less than in the original BCM rule.
Caitlyn R. Witkowski, Marcel T.J. van der Meer, Brian Blais, Jaap S. Sinninghe Damsté, and Stefan Schouten. Algal biomarkers as a proxy for pCO$_2$: Constraints from late Quaternary sapropels in the eastern Mediterranean. Organic Geochemistry. Volume 150, December 2020.
Records of carbon dioxide concentrations (partial pressure expressed as pCO$_2$) over Earth's history provide trends that are critical to understand our changing world. To better constrain pCO$_2$ estimations, here we test organic pCO$_2$ proxies against the direct measurements of pCO$_2$ recorded in ice cores. Based on the concept of stable carbon isotopic fractionation due to photosynthetic CO$_2$ fixation (Ɛp), we use the stable carbon isotopic composition (δ^{13}$C) of the recently proposed biomarker phytol (from all photoautotrophs), as well as the conventionally used alkenone biomarkers (from specific species) for comparison, to reconstruct pCO$_2 over several Quaternary sapropel formation periods (S1, S3, S4, and S5) in the eastern Mediterranean Sea. The reconstructed pCO$_2$ values are within error of the ice core values but consistently exceed the ice core values by ca. 100 µatm. This offset corresponds with atmospheric disequilibrium of present day CO$_2$[aq] concentrations in the Mediterranean Sea from global pCO$_2$, equivalent to ca. 100 µatm, although pCO$_2$ estimates derived from individual horizons within each sapropel do not covary with the ice core values. This may possibly be due to greater variability in local CO$_2$[aq] concentration changes in the Mediterranean, as compared with the global average pCO$_2$, or possibly due to biases in the proxy, such as variable growth rate or carbon-concentrating mechanisms. Thus, the offset is likely a combination of physiological or environmental factors. Nevertheless, our results demonstrate that alkenone- and phytol-based pCO$_2$ proxies yield statistically similar estimations (P-value = 0.02, Pearson's r-value = 0.56), and yield reasonable absolute estimations although with relatively large uncertainties (±100 µatm).
Blais, Brian S. Model Comparison in the Introductory Physics Laboratory. The Physics Teacher 58.3 (2020): 209-213.
Model comparison is at the heart of all scientific methodologies. Progress is made in science by constructing many models (possibly of different complexities), testing them against measurements, and determining which of them explain the data the best. It is my observation, however, that in many introductory physics labs we provide students with the materials and methods to verify the “correct” model of the experiment they are performing, e.g. measuring “g” or verifying the period of a pendulum. In this way, we do our students a disservice and don't allow them to experience the richness and creativity that constitutes the scientific enterprise. Limiting the lab to the “correct” model can have its uses—for example, getting the students to practice the proper methods to measure lengths and times or to support the specific theory covered in the lecture portion of the class. However, when students perform these labs, they come to view these activities as repetitive and mechanical, reinforcing the notion that science concerns not the true exploration of nature but simply the verification of what we already know. By verifying what we already know, the laboratory experience does not improve overall understanding and can mislead students about the methods of science overall.
This study applies dynamical and statistical modeling techniques to quantify the proliferation and popularity of trending hashtags on Twitter. Using time-series data reflecting actual tweets in New York City and San Francisco, we present estimates for the dynamics (i.e., rates of infection and recovery) of several hundred trending hashtags using an epidemic modeling framework coupled with Bayesian Markov Chain Monte Carlo (MCMC) methods. This methodological strategy is an extension of techniques traditionally used to model the spread of infectious disease. We demonstrate that in some models, hashtags can be grouped by infectiousness, possibly providing a method for quantifying the trendiness of a topic.
Mathematical models of epidemic dynamics offer significant insight into predicting and controlling infectious diseases. The dynamics of a disease model generally follow a susceptible, infected, and recovered (SIR) model, with some standard modifications. In this paper, we extend the work of Munz et.al (2009) on the application of disease dynamics to the so-called ``zombie apocalypse'', and then apply the identical methods to influenza dynamics. Unlike Munz et.al (2009), we include data taken from specific depictions of zombies in popular culture films and apply Markov Chain Monte Carlo (MCMC) methods on improved dynamical representations of the system. To demonstrate the usefulness of this approach, beyond the entertaining example, we apply the identical methodology to Google Trend data on influenza to establish infection and recovery rates. Finally, we discuss the use of the methods to explore hypothetical intervention policies regarding disease outbreaks.
In this paper, we explore a variety of models attempting to explain the pollution-income relationship (PIR). There has been much literature addressing the notion of an environmental Kuznets curve (EKC). Many researchers find an EKC relationship for certain pollutants, while others do not find evidence of an EKC relationship. There is also literature formally critiquing the EKC. We employ cross-sectional, panel, and time-series analysis to add insight into the relationship between economic growth and environmental degradation, a research area that is far from consensual and that has practical implications. We ultimately find that the clearest case of an EKC effect in our study arises in the analysis of organic water pollution, while there is modest evidence suggesting an EKC effect with regard to CO$_2$, NO, and methane. We also present ample evidence suggesting an anti-EKC effect for PM10. Our analysis causes us to question the existence of an EKC effect throughout the environment in general.
We measured I'C and I'15N values and carbon and nitrogen elemental concentrations of leaves collected from Metasequoia glyptostroboides Hu et Cheng trees cultivated at 39 sites across the United States under different latitudes and climatic regions. I'D values from south facing leaf n-alkanes of 27 trees were also determined. Climate data over the past 50 years (1950a2009) were compiled from stations near each site. Isotope data were cross plotted against each geographic and climatic parameter, including latitude, annual mean temperature (AMT), spring (FebruaryaMay) mean temperature (SMT), annual mean precipitation (AMP), and spring mean precipitation (SMP). Statistical analyses revealed the following signicant correlations: 1) a strong negative correlation between n-alkane D and latitude; 2) statistically signicant correlations between D and both AMT and SMT; 3) a weaker but still signicant correlation between I'D and SMP; 4) statistically signicant relationships between carbon concentration and both temperature and precipitation parameters, especially AMP; 5) an unexpected correlation between nitrogen concentration and SMP. These results bear strong implications for using I'13C and I'D values obtained from fossil Metasequoia as paleoclimatic and paleoenvironmental proxies.
It is often challenging, especially at the beginning of a course, to find good examples where students can actively explore and grapple with the methods of science. We want them to learn the connection between observation, theory, prediction, evidence, and falsification, but to really accomplish this we need platforms for which the students are able to design and implement experiments, and we need to be able to see the results of those experiments relatively quickly. There are some nice ideas using games1 and simple demonstrations and labs.2,3 I have found an example that is both entertaining for the students and rich enough in behavior to be an ideal platform for introducing scientific thinking: the automatic flushing toilet (Fig. 1).
LEGO MINDSTORMSA(r) NXT (Lego Group, 2006) is a perfect platform for introducing programming concepts, and is generally targeted toward children from age 8-14. The language which ships with the MINDSTORMSA(r), called NXTg, is a graphical language based on LabVIEW (Jeff Kodosky, 2010). Although there is much value in graphical languages, such as LabVIEW, a text-based alternative can be targeted at an older audiences and serve as part of a more general introduction to modern computing. Other languages, such as NXC (Not Exactly C) (Hansen, 2010) and PbLua (Hempel, 2010), fit this description. Here we introduce PyNXC, a subset of the Python language which can be used to program the NXT MINDSTORMSA(r). We present results using PyNXC, comparisons with other languages, and some challenges and future possible extensions.
Blais, B.S, Cooper, L.N , and Shouval H.Z. 2008. Effect of correlated lateral geniculate nucleus firing rates on predictions for monocular eye closure versus monocular retinal inactivation Physical Review E 80 (6): 061915.
Monocular deprivation experiments can be used to distinguish between different ideas concerning properties of cortical synaptic plasticity. Monocular deprivation by lid suture causes a rapid disconnection of the deprived eye connected to cortical neurons whereas total inactivation of the deprived eye produces much less of an ocular dominance shift. In order to understand these results one needs to know how lid suture and retinal inactivation affect neurons in the lateral geniculate nucleus (LGN) that provide the cortical input. Recent experimental results by Linden et al. showed that monocular lid suture and monocular inactivation do not change the mean firing rates of LGN neurons but that lid suture reduces correlations between adjacent neurons whereas monocular inactivation leads to correlated firing. These, somewhat surprising, results contradict assumptions that have been made to explain the outcomes of different monocular deprivation protocols. Based on these experimental results we modify our assumptions about inputs to cortex during different deprivation protocols and show their implications when combined with different cortical plasticity rules. Using theoretical analysis, random matrix theory and simulations we show that high levels of correlations reduce the ocular dominance shift in learning rules that depend on homosynaptic depression (i.e., Bienenstock-Cooper-Munro type rules), consistent with experimental results, but have the opposite effect in rules that depend on heterosynaptic depression (i.e., Hebbian/principal component analysis type rules).
Ocular dominance (OD) plasticity is a robust paradigm for examining the functional consequences of synaptic plasticity. Previous experimental and theoretical results have shown that OD plasticity can be accounted for by known synaptic plasticity mechanisms, using the assumption that deprivation by lid suture eliminates spatial structure in the deprived channel. Here we show that in the mouse, recovery from monocular lid suture can be obtained by subsequent binocular lid suture but not by dark rearing. This poses a significant challenge to previous theoretical results. We therefore performed simulations with a natural input environment appropriate for mouse visual cortex. In contrast to previous work we assume that lid suture causes degradation but not elimination of spatial structure, whereas dark rearing produces elimination of spatial structure. We present experimental evidence that supports this assumption, measuring responses through sutured lids in the mouse. The change in assumptions about the input environment is sufficient to account for new experimental observations, while still accounting for previous experimental results.
BCM (Bienenstock et al., 1982) refers to the theory of synaptic modification first proposed by Elie Bienenstock, Leon Cooper, and Paul Munro in 1982 to account for experiments measuring the selectivity of neurons in primary sensory [8]cortex and its dependency on neuronal input. It is characterized by a rule expressing synaptic change as a Hebb-like product of the presynaptic activity and a nonlinear function, \phi(y;\theta_M) , of postsynatic activity, y. For low values of the postsynaptic activity ( y<\theta_M ), \phi is negative; for y>\theta_M , \phi is positive. The rule is stabilized by allowing the modification threshold, \theta_M , to vary as a super-linear function of the previous activity of the cell. Unlike traditional methods of stabilizing Hebbian learning, this "sliding threshold" provides a mechanism for incoming patterns, as opposed to converging afferents, to compete. A detailed exploration can be found in the book Theory of Cortical Plasticity (Cooper et al., 2004). For an open-source implementation of the BCM, amongst other synaptic modification rules, see the Plasticity package[1] .
In the present work we introduce the problem of determining the probability that a rotating and bouncing cylinder (i.e. flipped coin) will land and come to rest on its edge. We present this problem and analysis as a practical, nontrivial example to introduce the reader to Bayesian model comparison. Several models are presented, each of which take into consideration different physical aspects of the problem and the relative effects on the edge landing probability. The Bayesian formulation of model comparison is then used to compare the models and their predictive agreement with data from hand-flipped cylinders of several sizes.
Our collaborative research proposes to study the synaptic and cellular basis of receptive field plasticity in visual cortex. Recently some of our efforts have concentrated on accounting for novel aspects of cellular responses and plasticity observed in the visual cortex of the rat. In recently published findings we provided evidence that pairing visual cues with subsequent rewards in awake behaving animals results in the emergence, in the primary visual cortex (V1), of reward-timing activity (Shuler and Bear, 2006). Further, the properties of reward-timing activity suggest that it is generated locally within V1, implying that V1 is privy to a signal relating the acquisition of reward. We provide here a model demonstrating how such interval timing of reward could emerge in V1. A fundamental assumption of this work is that the timing characteristics of the V1 network are encoded in the lateral connectivity within the network, consequently the plasticity assumed in this model is of these recurrent connections. Using this assumption, no prior stimulus-locked temporal representation is necessary. The plasticity of recurrent connections is implemented through an interaction between an activity dependent Hebbian like plasticity, and a neuromodulatory signal signifying reward. It is demonstrated that such a global reinforcement signal is sufficient for interval time learning by stabilizing changes in nascent synaptic efficacy resultant from prior visually-evoked activity. By modifying synaptic weight change within the recurrent network of V1, our model transforms temporally restricted visual events into neurally persistent activity relating to their associated reward timing expectancy.
Synaptic plasticity is a likely basis for information storage by the neocortex. Understanding cortical plasticity requires coordinated investigation of both underlying cellular mechanisms and their systems-level consequences in the same model system. However, establishing connections between the cellular and system levels of description is non-trivial. A major contribution of theoretical neuroscience is that it can link different levels of description, and in doing so can direct experiments to the questions of greatest relevance. The objective of the current project is to generate a theoretical description of experience-dependent plasticity in the rodent visual system. The advantages of rodents are, first, that knowledge of the molecular mechanisms of synaptic plasticity is relatively mature and continues to be advanced with genetic and pharmacological experiments, and second, rodents show robust receptive field plasticity in visual cortex (VC) that can be easily and inexpensively monitored with chronic recording methods. The project aims are threefold. First, the activity of inputs to rat visual cortex will be recorded in different viewing conditions that induce receptive field (RF) plasticity, and these data will be integrated into formal models of synaptic plasticity. Second, the dynamics of RF plasticity will be simulated using existing spike rate-based algorithms and compared will experimental observations. Third, the consequences of new biophysically plausible plasticity algorithms, based on spike timing and metaplasticity, will be analyzed and compared with experiments.6
To examine climatic signals registered as carbon isotopic values in leaf tissues of C3 plants, we collected mature leaf tissues from northern and southern leaves from the 1947 batch of Metasequoia trees planted along a latitudinal gradient of the United States. Samples from 40 individual trees, along with fossilized material from the early Tertiary of the Canadian Arctic, were analyzed for C and N concentration and isotopic values. After the removal of free lipids, EA-IRMS was used to measure both concentration and bulk C and N isotopic values on leaf residues. The generated datasets were then merged with climate data compiled from each tree site recorded as average values over the past thirty years (1971-2002, NOAA database). When the isotope data were cross plotted against each geographic and climatic indicator, Latitude, Mean Annual Temperature (MAT), Average Summer Mean Temperature (ASMT)(June-August), Mean Annual Precipitation (MAP), and Average Summer Mean Precipitation (ASMP) respectively correlation patterns were revealed. The best correlating trend was obtained between temperature parameters and C isotopic values, and this correlation is stronger in the northern leaf samples than the southern samples. The Nitrogen data remained inconclusive as the result of the N isotope signals being site specific. This investigation represents a comprehensive examination on climatic signals registered as C isotopic values on a single species that is marked by single genetic source. The results bear implications on paleoclimatic interpretations of C isotopic signals obtained from fossil plant tissues.
Rate-based neuron models have been successful in understanding many aspects of development such as the development of orientation selectivity(Bienenstock et al., 1982; Oja, 1982; Linsker, 1986; Miller, 1992; Bell and Sejnowski, 1997), the particular dynamics of visual deprivation(Blais et al., 1999) and the development of direction selectivity(Wimbauer et al., 1997; Blais et al., 2000). These models do not address phenomena such as temporal coding, spike-timing dependant synaptic plasticity, or any short-time behavior of neurons. More detailed spiking models (Song et.al, 2000; Shouval et.al. 2002; Yeung et.al. 2004) address these issues, and have had some success, but have failed to develop receptive fields in natural environments. These more detailed models are diffiult to explore, given their large number of parameters and the run-time computational limitations. In addition, their results are often diffiult to compare directly with the rate-based models. We propose a model, which we call a spiking-rate model, which can serve as a middle-ground between the over simplistic rate-based models, and the more detailed spiking models. The spiking-rate model is a spiking model where all of the underlying processes are continuous Poisson, the summation of inputs is entirely linear (although non-linearities can be added), and the generation of outputs is done by calculating a rate output and then generating an appropriate Poisson spike train. In this way, the limiting behavior is identical to a rate-based model, but the proper ties of spiking models can be incorporated more easily. We present the development of receptive i!elds with this model in various visual environments. We then present the necessary conditions for the receptive field development in the spiking-rate models, and make comparisons to detailed spiking models, in order to more clearly understand the necessary conditions for receptive field development
Modifications in the strengths of synapses are thought to underlie memory, learning, and development of cortical circuits. Many cellular mechanisms of synaptic plasticity have been investigated in which differential elevations of postsynaptic calcium concentrations play a key role in determining the direction and magnitude of synaptic changes. We have previously described a model of plasticity that uses calcium currents mediated by N-methyl-D-aspartate receptors as the associative signal for Hebbian learning. However, this model is not completely stable. Here, we propose a mechanism of stabilization through homeostatic regulation of intracellular calcium levels. With this model, synapses are stable and exhibit properties such as those observed in metaplasticity and synaptic scaling. In addition, the model displays synaptic competition, allowing structures to emerge in the synaptic space that reflect the statistical properties of the inputs. Therefore, the combination of a fast calcium-dependent learning and a slow stabilization mechanism can account for both the formation of selective receptive fields and the maintenance of neural circuits in a state of equilibrium.
This invaluable book presents a theory of cortical plasticity and shows how this theory leads to experiments that test both its assumptions and consequences. It ellucidates, in a manner that is accessible to students as well as researchers, the role which the BCM theory has played in guiding research and suggesting experiments that have led to our present understanding of the mechanisms underlying cortical plasticity. Most of the connections betwen theory and experiment that are discussed require complex simulations. A unique feature of the book is the accompanying software package, Plasticity . This is provided complete with source code, and enables the reader to repeat any of the simulations quoted in the book as well as to vary either parameters or assumptions. *Plasticity * is thus a research and an educational tool. Readers can use it to obtain hands-on knowledge of the structure of BCM and various other learning algorithms. They can check and replicate our results as well as test algorithms and refinements of their own.
The idea of energy balance used to explain the greenhouse effect and global warming is often confusing for students, primarily because the standard quantitative analysis uses many constants and units. A "round" unit method is presented, which maintains the quantitative aspects of the standard analysis, but is much more intuitive for students.
This article describes the construction of a safe, programmable, automatic thermal cycler for PCR that can be easily constructed by persons with basic soldering and mechanical skills for under $25 in parts and a modest computer such as IBM 486, all of which are readily available. The cycler relies on the heating provided by an incandescent light bulb and cooling by simple convection.
The sign and magnitude of bi-directional synaptic plasticity have been shown to depend on: the rate of presynaptic stimulation, the level of postsynaptic depolarization, and the precise relative timing between pre and postsynaptic spikes. It has been proposed that these different induction paradigms can coexist, and be accounted for by a single learning rule that depends on the dynamics of intracellular calcium concentration. We extend this rule to a multi-synaptic environment, where collective properties such as cooperativity, competition and selectivity can be investigated.
A unified, biophysically motivated Calcium-Dependent Learning model has been shown to account for various rate-based and spike time-dependent paradigms for inducing synaptic plasticity. Here, we investigate the properties of this model for a multi-synapse neuron that receives inputs with different spike-train statistics. In addition, we present a physiological form of metaplasticity, an activity-driven regulation mechanism, that is essential for the robustness of the model. A neuron thus implemented develops stable and selective receptive fields, given various input statistics
Recently, a new property of synaptic plasticity, named synaptic scaling, has been described (Turrigiano et al., 1998). Synaptic scaling is an activity-dependent physiological mechanism for preserving cortical homeostasis. Its underlying biophysical basis, however, is not yet elucidated. Homeostatic metaplasticity had previously been proposed as a means of stabilizing the inherently unstable Hebbian plasticity. For example, the BCM theory of synaptic plasticity assumes a sliding modification threshold that stabilizes learning while producing selective receptive fields (Bienenstock et al., 1982). Here, we suggest a biophysical formulation of metaplasticity as the underlying mechanism for synaptic scaling. We have recently developed a unified theory of synaptic plasticity that can account for various induction paradigms (Shouval et al., 2002). In this model, AMPA receptor plasticity is induced by calcium transients through NMDA receptors. Thus changing the properties of NMDA receptors can alter the form of synaptic plasticity. We propose a form of metaplasticity that is based on cell-wide activity-dependent regulation of NMDA receptor conductance. Simulations of this combined plasticity-metaplasticity system show that, as the average input rate increases, synaptic weights decay, and the resulting distribution of synaptic weights is unimodal. In addition, the output-firing rate is roughly maintained, even in the absence of hard constraints on individual or the average synaptic weights. Therefore, metaplasticity and synaptic plasticity can account for the experimental observations of synaptic scaling and maintain cortical homeostasis. Additional mechanisms that explicitly enforce scaling are not required. Support Contributed By: the Brown University Brain Science Program and the Burroughs-Wellcome Fund
Intracellular calcium concentration has been proposed as the key associative signal for the Hebbian synaptic plasticity. The Unified Calcium Model has been able to account for various plasticity induction protocols, such as rate-based and spike time-dependent plasticity. Here, we investigate the properties of this model in a multi-synapse neuron receiving inputs with different spatiotemporal spike train statistics. In addition, we present a physiological form of metaplasticity, an activity-driven robustness-inducing regulation mechanism. A neuron thus implemented is stable and spontaneously develop selectivity to a subset of the stimulating inputs.
A unified, biophysically motivated Calcium-Dependent Learning model has been shown to account for various rate-based and spike time-dependent paradigms for inducing synaptic plasticity. Here, we investigate the properties of this model for a multi-synapse neuron that receives inputs with different spike-train statistics. In addition, we present a physiological form of metaplasticity, an activity-driven regulation mechanism, that is essential for the robustness of the model. A neuron thus implemented develops stable and selective receptive fields, given various input statistics
We present a simulation environment, Plasticity , which allows the user to perform a wide variety of simulations of rate-based neural networks. The package focuses on, but is not limited to, simulations of visual neurons and allows one to compare on equal footing many of the most common learning rules. This includes variants of Hebbian learning( Linsker, 1986; MacKay and Miller, 1994; Erwin and Miller, 1998), BCM(Bienenstock et al., 1982; Intrator and Cooper, 1992; Blais et al., 1999), and ICA(Hyvarinen and Oja, 1997; Blais et al., 1998). The goal of this package is consistent with the philosophy of reproducible research: providing a complete package which can fully reproduce any results or figures in publications.(Schwab et al., 2000). One aspect of this package which makes it different than other neural network packages is that it allows the user to explore environments from simplified low dimensional vectors(Clothiaux et al., 1991), to high dimensional correlation-based environments( Erwin and Miller, 1998) to natural images(Blais et al., 1998). The package is currently being extended to include spike-based learning.
The sign and magnitude of bi-directional synaptic plasticity have been shown to depend on: the rate of presynaptic stimulation, the level of postsynaptic depolarization, and the precise relative timing between pre and postsynaptic spikes. It has been proposed that these different induction paradigms can coexist, and be accounted for by a single learning rule that depends on the dynamics of intracellular calcium concentration. We extend this rule to a multi-synaptic environment, where collective properties such as cooperativity, competition and selectivity can be investigated.
Different mechanisms that could form the molecular basis for bi-directional synaptic plasticity have been identified experimentally and corresponding biophysical models can be constructed. However, such models are complex and therefore it is hard to deduce their consequences to compare them to existing abstract models of synaptic plasticity. In this paper we examine two such models:a phenomenological one inspired by the phenomena of AMPA receptor insertion, and a more complex biophysical model based on the phenomena of AMPA receptor phosphorylation. We show that under certain approximations both these models can be mapped on to an equivalent, calcium-dependent, differential equation. Intracellular calcium concentration varies locally in each postsynaptic compartment, thus the plasticity rule we extract is a single-synapse rule. We convert this single synapse plasticity equation to a multi- synapse rule by incorporating a model of the NMDA receptor. Finally we suggest a mathematical embodiment of metaplasticity, which is consistent with observations on NMDA receptor properties and dependence on cellular activity. These results, in combination with some of our previous results, produce converging evidence for the calcium control hypothesis including a dependence of synaptic plasticity on the level of intercellular calcium as well as on the temporal pattern of calcium transients.
The receptive fields for simple cells in visual cortex show a strong preference for edges of a particular orientation and display adjacent excitatory and inhibitory subfields. These subfields are projections from ON-center and OFF-center lateral geniculate nucleus cells, respectively. Here we present a single-cell model using ON and OFF channels, a natural scene environment, and synaptic modification according to the Bienenstock, Cooper, and Munro (BCM) theory. Our results indicate that lateral geniculate nucleus cells must act predominantly in the linear region around the level of spontaneous activity, to lead to the observed segregation of ON/OFF subfields.
Most simple and complex cells in the cat striate cortex are both orientation and direction selective. In this paper we use single cell learning rules to develop both orientation and direction selectition and direction selectivity in a natural scene environment. We show that a simple PCA rule is inadequate for developing direction selectivity, but that the BCM rule as well as similar higher order rules can. We also demonstrate that the convergence of lagged and non-lagged cells depends on the velocity of motion in the environment, and that strobe rearing disrupts this convergence resulting in a loss of direction selectivity.
We offer an explanation of the pre-eye-opening development of four properties of the mammalian visual system: cortical orientation selectivity, the localized nature of retinogeniculate connections, the retinotopic map in the LGN, and the eye-specific lamination of the LGN. We investigate the possibility that the development of these properties results from structure in the activity of the prenatal visual environment. Three separate approaches are taken to modeling this activity. We model the prenatal visual environment as consisting of either: explicitly correlated noise, retinally processed noise, or retinal waves. A study oretinal waves. A study of the behavior of the BCM and PCA learning rules in these model environments leads to an understanding of the emergence of the four visual system properties. Both rules are consistent with the initial development of orientation selectivity, but, unlike BCM, the PCA rule is unable to account for the development of the LGN properties.
Visual cortical simple cells show a strong preference for edges of a particular orientation[Hubel and Wiesel,1962]. The receptive field of the cortical cell shows adjacent excitatory and inhibitory subfields, which are projections from ON-center and OFF-center LGN cells, respectively[Reid and Alonso,1995]. Here we present a single cell model using ON and OFF channels, a natural scene environment, and the BCM[Bienenstock et al.,1982] learning rule. The results from the model imply that the input distribution from LGN to cortex should be almost symmetrical in order to develop the proper segregation of ON/OFF subfields; There is a relation between the organization of simple cell receptive fields and the shape ofelds and the shape of the input distribution.
Although there have been extensive investigations in computational neuroscience, the opportunity (that has made such a marked difference in physical sciences) to test detailed and subtle quantitative consequences of a theory against experimental results is rare. In this paper we outline a testable consequence of two contrasting theories of synaptic plasticity applied to the disconnection in visual cortex of the closed eye in monocular deprivation (MD). This disconnection is sometimes thought to be the consequence of a process that stems from a competition of inputs for a limited resource such as neurotrophin. Such a process leads to what we call spatial competition, or heterosynaptic synaptic modification. A contrasting view, exemplified by the Bienenstock, Cooper, and Munro (BCM) theory, is that patterns of input activity compete in the temporal domain. This temporal competition is homosynaptic and does not require a conserved resource. The two erved resource. The two mechanisms, homosynaptic and heterosynaptic, are the distinguishing characteristics of two general classes of learning rules which we explore, using a realistic environment composed of natural scenes. These alternative views lead to opposite dependence on the level of presynaptic activity of the rate of disconnection of the closed eye in monocular deprivation. This strong and testable consequence sets the stage for a critical distinguishing experiment; the experiment has been done and supports the second view. These results have important implications for the processes of learning and memory storage in neocortex.
It is widely believed that much of learning, memory storage, and resulting organization of many parts of the brain occur due to the modification of the efficacy or strength of at least some of the synaptic junctions between neurons. The genetic code is not large enough to specify the strength of all of the synapses in the brain, so it is more reasonable to assume that there are some general mechanisms for modifying the synapses of a neuron based on the input signals to that neuron. In nals to that neuron. In this way the neuron becomes adaptive to the structure in the input patterns, or the environment. The environment, therefore, plays a crucial role in the formation of the general properties of neurons and may give us a significant insight into the formation of memory. The central theme of this work is role of the environment in the development, and maintenance, of orientation selectivity and ocular dominance in the visual cortex. We compare statistically and biologically motivated learning rules in a realistic model of the input environment, consisting of natural scene images. From simulations and analysis we are able to propose experiments, some of which are currently being performed, in order to distinguish between different synaptic modification rules, which in turn allows us to get a glimpse at some of the possible underlying mechanisms. An understanding of the properties of the learning rules, in relation to the environment, allows us to propose a simplified environment which is analytically tractable. This environment captures some of the qualitative features of the natural scene images, but provides a better understanding of the role of the environment in learning. Other modifications of the input environment, some motivated by biology, are also presented. These modifications are beginning to give the first indications of some of the subtle effects of the environment: effects which can have a profound impact on the response properties of neurons and the organization of many parts of the brain.
Most simple cells in the cat striate cortex are both orientation and direction selective. In this paper we use single cell learning rules to develop both orientation and direction selectivity in a natural scene environment. We show that a simple PCA rule is inadequate to develop direchow that a simple PCA rule is inadequate to develop direction selectivity, but that the BCM rule and similar rules can. We compare these models to experiments in motion deprived environments, such as strobe rearing, and show some connections between the development of direction and orientation selectivity.
We study several statistically and biologically motivated learning rules using the same visual environment, one made up of natural scenes, and the same single cell neuronal architecture. This allows us to concentrate on the feature extraction and neuronal coding properties of these rules. Included in these rules are kurtosis and skewness maximization, the quadratic form of the BCM learning rule, and single cell ICA. Using a structure removal method, we demonstrate that receptive fields developed using these rules depend on a small portion of the distribution. We find that the quadratic form of the BCM rule behaves in a manner similar to a kurtosis maximization rule when the distribution contains kurtotic directions, although the BCM modification equatioCM modification equations are computationally simpler.
We study several statistically and biologically motivated learning rules using the same visual environment and neuronal architecture. This allows us to concentrate on the feature extraction and neuronal coding properties of these rules. We i!nd that the quadratic form of the BCM rule behaves in a manner similar to a kurtosis maximization rule when the distribution contains kurtotic directions, although the BCM modii!cation equations are computationally simpler.
Why is it that physicists would choose to study the brain, a topic which one usually associates with biology? Why choose this rather than pursue more traditional lines of research? Do physicists have something to offer to the field which others have lacked? These questions force us to look closely at the process of science, and how physicists like to look at the world.
Receptive fields in the visual cortex can be altered by changing the visual environment, as has been shown many times in deprivation experiments. In this paper we simulate this set of experiments using two different models of cortical plasticity, BCM and PCA. The visual environment used is composed of natural images for open eye and of noise for closed eyes. We measure the response of the neurons to oriented stirse information of the neuronal response to provide a preliminary quantitative comparison between the cortical models and experiment.
The Noise Sensitivity Signature (NSS), originally introduced by Grossman and Lapedes (1993), was proposed as an alternative to cross validation for selecting network complexity. In this paper, we extend NSS to the general problem of regression estimation. We also present results from regularized linear regression simulations which indicate that for problems with few data points, NSS regression estimates perform better than Generalized Cross Validation (GCV) regression estimates [Wahba90].